- Published on
Introduction to Linear Regression
12 min read
- Authors
- Name
- Kiarash Soleimanzadeh
- https://go.kiarashs.ir/twitter
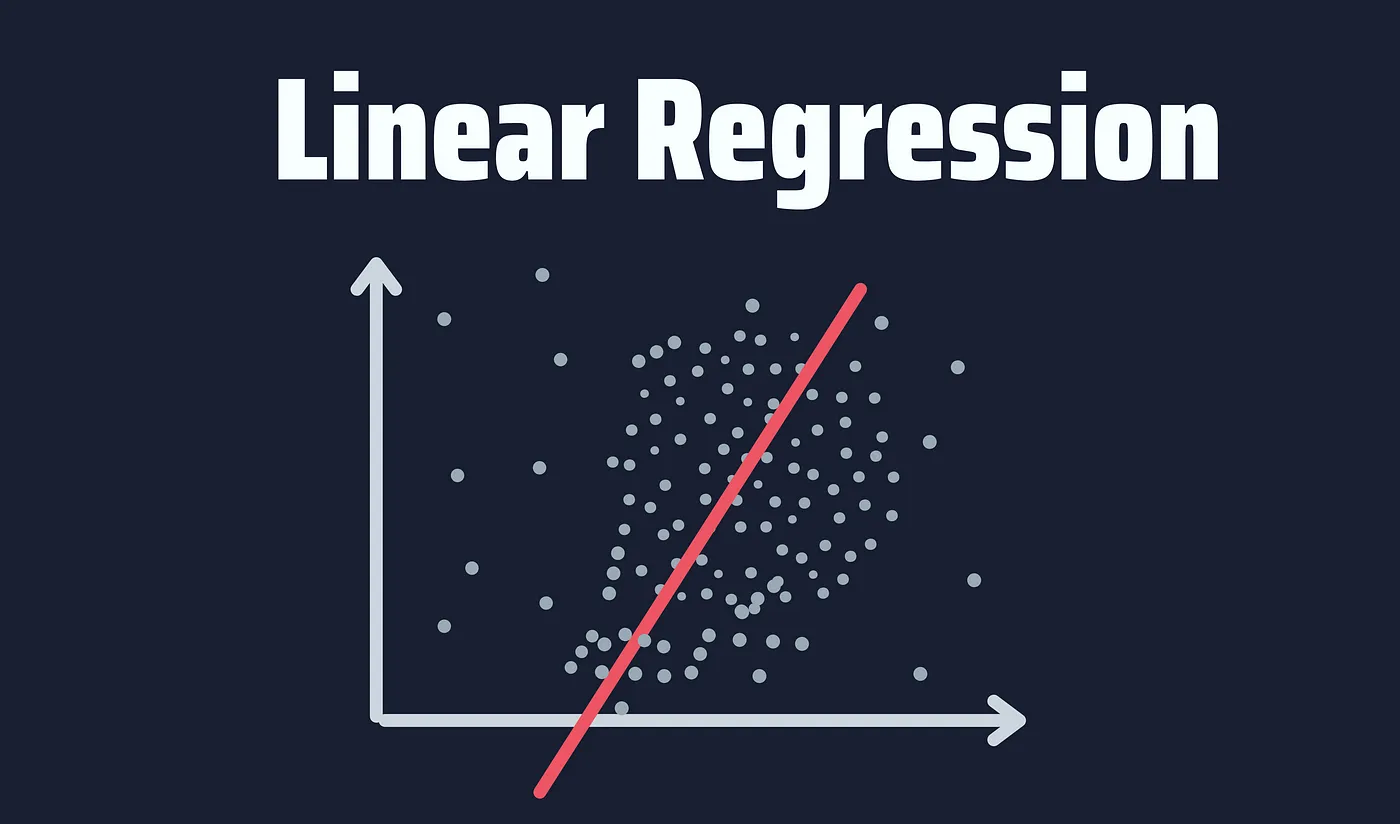
Table of Contents
Linear regression is among the most widely used tools in machine learning. Linear models are linear simply because the outputs are modeled as linear combinations of input vectors. Hence, we want to learn a function that describes with as little error as possible, the linear relationship between inputs and outputs.
Model definition
Consider a matrix of inputs , a vector of weights , and output vector . We predict given as:
Where is the bias or intercept. Note we add a "hat" to the unknown estimated parameters to distinguish them from known given values. To express a linear regression in matrix notation, we can incorporate a constant vector to , and the bias into the vector of weights , to obtain:
Note that in the absence of the bias term, our solution will be forced to pass through the origin of the coordinate space, forming a subspace. Adding the bias term allows our solution to be detached of such constrain, forming an affine set.
Cost function
The goal of our model is to find a set of weights that minimizes some measure of error or cost function. The most popular measure of error is the Sum of Squared Errors (SSE), sometimes referred to as Residual Sum of Squares (RSS). The expression describing the SSE is:
In words: we take the squared difference between the target and predicted value for the row of and sum up the result. Again, we can express this in matrix notation as:
In machine learning is common to take the mean of the SSE to obtain the Mean Squared Error (MSE) as:
Model training
Given that the SSE is a quadratic function of the weights, the error surface is convex, hence always has a minimum. There are multiple ways to adjust the weights to minimize our measure of error. One way, know as closed-form solution or normal equations, is to solve for where the derivatives with respect to the weights are :
Using the matrix notation, we solve for as:
Note that the solution works only if is nonsingular or invertible (see here). Geometrically, this means that each vector in is independent of each other. Otherwise, we can compute the minimum norm solution for the singular case (see here). If you are familiar with iterative methods, you can think of the closed-form solution as a one-step solution.
A different approach to solve a linear model with iterative methods like gradient descent. This method is preferred when the matrix is large, as inverting large matrices is computationally expensive. I won't describe gradient descent in this section (which you can review here), to maintain our focus in the linear regression problem.
Simple linear regression example
Let's try out a simple linear regression example with Python and sklearn. By simple, I mean one feature and one output. In the next section will do a multivariable or multi-feature regression.
We will load the Boston house prices dataset from sklearn. This dataset contains 506 rows (houses), 13 columns (houses features). The targets (house prices) range from 5 to 50. Our goal is just to show how to run a linear regression with sklearn, so we won't do an exploratory data analysis this time. A detailed description of the dataset attributes can be found here.
# Libraries for this section
from sklearn.datasets import load_boston
from sklearn import linear_model
from sklearn.metrics import mean_squared_error, r2_score
from sklearn.model_selection import train_test_split
import numpy as np
import altair as alt
import pandas as pd
alt.themes.enable('dark')
# ThemeRegistry.enable('dark')
We first load the dataset using sklearn API
X, y = load_boston(return_X_y=True)
print(f'Dataset shape: {X.shape}')
# Dataset shape: (506, 13)
We split our data into training and testing sets, using a 80/20 split.
X_train, X_test, y_train, y_test = train_test_split(X, y, test_size = 0.2, random_state=0)
print(f'Training data shape: {X_train.shape}')
print(f'Testing data shape: {X_test.shape}')
print(f'Training labels shape: {y_train.shape}')
print(f'Testing labels shape: {y_test.shape}')
"""
Training data shape: (404, 13)
Testing data shape: (102, 13)
Training labels shape: (404,)
Testing labels shape: (102,)
"""
Since we want to run a regression with a single feature predictor, let's compute the correlation coefficients for each feature and the target (house prices).
corr = np.corrcoef(np.column_stack((X,y)).T)[:,13]
max_abs_corr = np.argmax(np.absolute(corr[0:13]))
print(f'correlation coefficient features and house prices: {np.round(corr, 2)}')
print(f'feature with max absolute correlation with house prices: {max_abs_corr}')
"""
correlation coefficient features and house prices: [-0.39 0.36 -0.48 0.18 -0.43 0.7 -0.38 0.25 -0.38 -0.47 -0.51 0.33
-0.74 1. ]
feature with max absolute correlation with house prices: 12
"""
Feature number 12 has the maximum absolute correlation with house prices, . According to the documentation, this is the % lower status of the population: the more low-status people around, the lower the house price.
Now we fit the model using the training set.
reg = linear_model.LinearRegression()
reg.fit(X_train[:, 12].reshape(-1, 1), y_train) # pick all the rows for the 12 variable
# LinearRegression(copy_X=True, fit_intercept=True, n_jobs=None, normalize=False)
The model has learned the coefficients or weights that best fit the data, which we can use to make predictions on the testing set.
y_pred = reg.predict(X_test[:, 12].reshape(-1, 1)) # pick all the rows for the 12 variable
We evaluate the overall performance by computing the SSE, MSE, and the coefficient of determination.
SSE = ((y_test - y_pred) ** 2).sum()
MSE = mean_squared_error(y_test, y_pred)
R2 = r2_score(y_test, y_pred)
print(f'Sum of Squared Errors: {np.round(SSE,2)}')
print(f'Mean of Squared Errors: {np.round(MSE,2)}')
print(f'R2 coefficient of determination: {np.round(R2,2)}')
"""
Sum of Squared Errors: 4726.3
Mean of Squared Errors: 46.34
R2 coefficient of determination: 0.43
"""
Based on a single feature, we obtain a , a , and a .
Finally, let's visualize the regression line for this pair of variables.
df = pd.DataFrame({'low-status-pop': X[:, 12], 'house-prices': y})
chart = alt.Chart(df).mark_point(color='fuchsia').encode(
x='low-status-pop',
y='house-prices')
chart + chart.transform_regression('low-status-pop', 'house-prices').mark_line(color='yellow')
Multivariable linear regression example
Now let's fit a model with all 13 features as predictors. For this we just need to remove [:, 12].reshape(-1, 1)
from the fit
and predict
methods.
multi_reg = linear_model.LinearRegression()
multi_reg.fit(X_train, y_train)
# LinearRegression(copy_X=True, fit_intercept=True, n_jobs=None, normalize=False)
y_pred_multi = multi_reg.predict(X_test)
Again, let's evaluate the overall performance by computing the SSE, MSE, and the coefficient of determination.
SSE_multi = ((y_test - y_pred_multi) ** 2).sum()
MSE_multi = mean_squared_error(y_test, y_pred_multi)
R2_multi = r2_score(y_test, y_pred_multi)
print(f'Sum of Squared Errors multivariable regression: {np.round(SSE_multi,2)}')
print(f'Mean of Squared Errors multivariable regression: {np.round(MSE_multi,2)}')
print(f'R2 coefficient of determination multivariable regression: {np.round(R2_multi,2)}')
"""
Sum of Squared Errors multivariable regression: 3411.8
Mean of Squared Errors multivariable regression: 33.45
R2 coefficient of determination multivariable regression: 0.59
"""
Based on the 13 features, we obtain a , a , and a . As expected, the error measures went down and the association measure went up, as more features provide more information for prediction.
Visualization is not possible for a 13 features regression, but you can make your best effort by imaging a 3D space and thinking "13!" with all your might.
References
Bishop, C. M. (2006). 3. Linear models for regression. Pattern recognition and machine learning. springer.
Deisenroth, M. P., Faisal, A. A., & Ong, C. S. (2020) 9. Linear Regression. In Mathematics for machine learning. Cambridge University Press.
Friedman, J., Hastie, T., & Tibshirani, R. (2009). 3. Linear Methods for Regression. In The elements of statistical learning (Vol. 1, No. 10). New York: Springer series in statistics.